In our first podcast, the CEO of H2K Labs, Heather Holst-Knudsen, talked about data and revenue with Chad Rose, the Managing Partner of Treehouse Technology Group, one of our technology partners. Chad started as a data engineer at S&P, where he learned how to build predictive models and manage data efficiently. With Phil West and Darton Rose, they founded Treehouse Technology Group in 2014 to serve the middle market's demand for better data and analytics. They identified a gap in the BI landscape and developed InsightOut to deliver enterprise-level analytics at an affordable cost. Heather noticed the same issues within the media, events, and business information industry. No data platform truly understood the complex business environment.
One-Sided vs. Two-Sided Business Models
While Chad’s experience has been focused on traditional business models, also known as one-sided businesses, there are some similar issues to the event, media, and business information industry. For example, if a SaaS business is focused on recurring revenue you need to monitor, you need to have your head around the metrics impacting that renewal churn, renewals, and AR. Those metrics are in and of themselves often very difficult to report on as so much of the data around that revenue stream sits in different sources. Another example is events, which really are little independent companies. The sales cycle depends on when the event is happening and works like an individual fiscal year you're managing. Finally, mergers and acquisitions are pretty common within the InsighOut customer base, and it's also common in media and events.
According to Chad, these businesses (media, events, and business information) “with so much in terms of data complexity, trying to meet the targets that they're trying to achieve, and with different business units each acting differently, make it harder to get a full picture of the entire business and just to get an ongoing pulse through an analytics implementation.” The data that's being generated is very much outside the CRM in many cases because of customer behaviors or all the people that are connecting with an event or a media customer.
In fact, each of the business units and the brands in those business units really operates like a mini business, with its own P&L, unique fiscal requirements, etc. The data that's being generated holds a lot of value which is being underutilized. It is very overwhelming in terms of figuring out where to start activating the data on the revenue side.
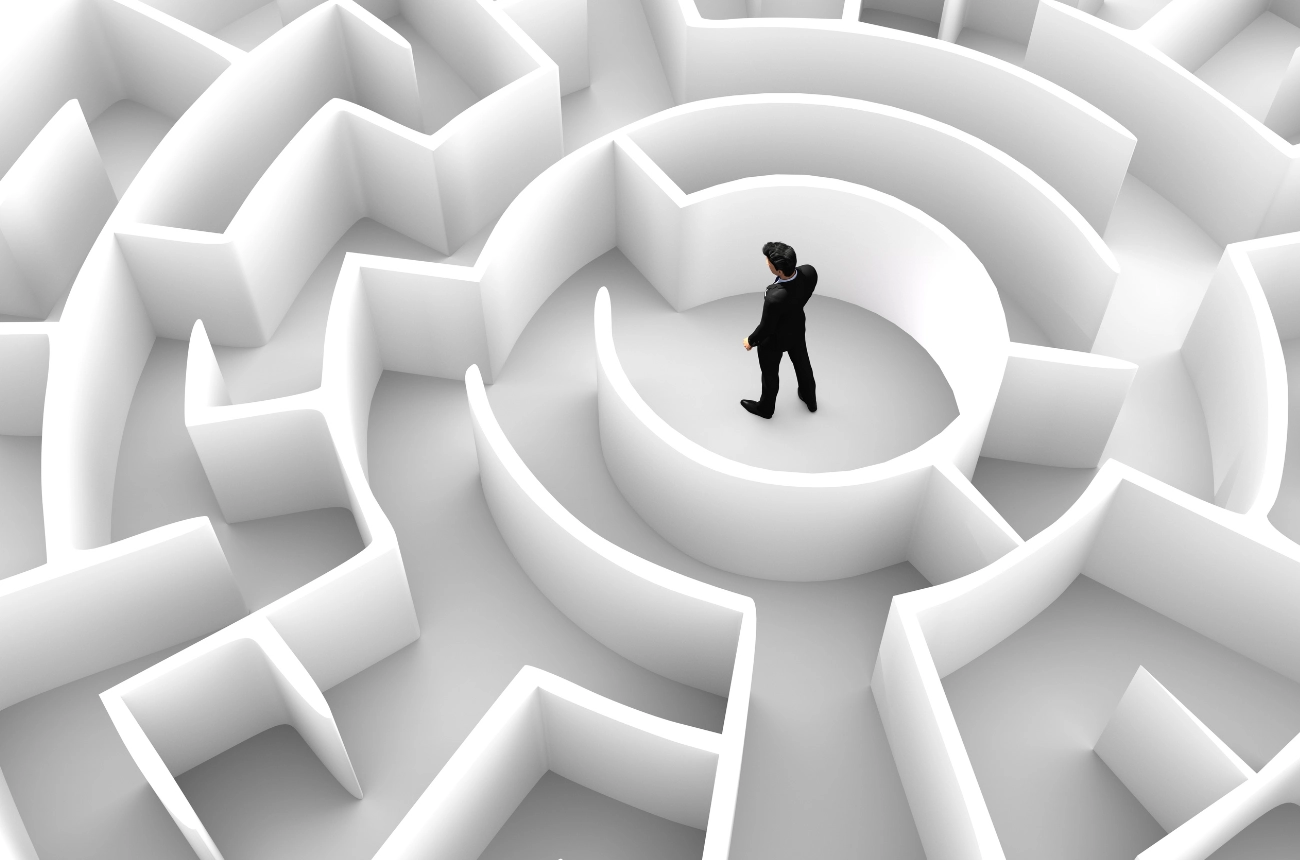
Challenges in developing an SSOT
A common misnomer is a lot of businesses feel they can't start an analytics initiative or think they can get anything out of the data. It is often viewed as a linear process where you have to get the data in shape and then be able to start reporting on it or be able to start getting value out of it. You can often do the two in parallel, and they actually reinforce each other if done properly.
Generally, the types of issues in creating an SSOT are not specific to media, events, and business information but are more around the data cleanliness and the data management practices within the organizations, or lack thereof. A lot of organizations are trying to get a better handle on how to input data and get the team, sales team, to input data consistently within the CRM.
However, revenue recognition is a big challenge unique to media, events, and business information. You have these products you're selling, and you have to deliver on what has been sold to recognize the revenue. Businesses need to have a handle on what has been delivered against what's been sold, booked but not yet delivered, those types of scenarios are within any business, but particularly in media, events, and business information; seem to be a pretty big challenge. You have to reconcile what's being done on the operational side against what's been done on the sales side and the financial side. Further, a recurring revenue business model can be pretty challenging, especially if you don't have good systems. Many media and events companies, want people to subscribe to a data product which adds even more complexity to revenue recognition. There hasn’t been a platform developed, until now, that can manage that data complexity.
Another challenge in the industry is product customization, for example, industry sectors they serve, like manufacturing, retail, food and beverage, or life sciences, and how their products are structured. Normalizing the data becomes more complex. These industries also have more systems than most where data might be stored; registration, lead scanning, and ad operations, just to name a few. So there are quite a few potential data sets, and those exist across a variety of systems and business units. It isn't critical to start with capturing all of the data when you're developing a single source of truth. But, "I think that within this space is pretty unique in that there's a lot of untapped potential within all those different data sets that if you can establish, it could be quite beneficial," according to Chad.
Simplifying the data landscape
The data landscape has changed dramatically, both in toolsets and organizational operations. It's gotten more complex as a business can have hundreds of tools being used for different needs, marketing systems, CRM, sales systems, and finance systems, but you might also have different systems managing your websites or different systems managing your customer engagement, and customer success. And so there are just more and more of those that each company is signing up for and using and leveraging.
From a user perspective, it is great as these tools are highly specialized, from a data perspective, it means data is siloed in many different systems and, therefore, highly unusable. Older business intelligence platforms are not designed to be flexible, you need to have a team of engineers to do the implementation and configuration. However, today, there are many more self-service platforms that are much easier to manage and use. Many of them have integrations using APIs or ways to automatically integrate, and many organizations do not leverage the connections.
Today business intelligence platforms are AI-enabled or becoming increasingly AI-enabled, and they are much faster to get to value and actual successful implementation. Additionally, some elements are even newer in terms of things like reverse ETL or write-backs, which enables businesses to push updates from the single source of truth, from your reporting system back into your source systems.
For the end user, you have aggregated all the data, and for example, you've highlighted some churn risks within the dataset, it is pushed back into the CRM, and the sales team gets notified and can action the issue. Until very recently, this was not possible, or you wouldn't be able to do it without having a big engineering team. As the systems have become open with their APIs, as the technology has become more AI-enabled, the result is organizations can achieve better results much faster, and far cheaper. And you can do so with the modern stack that exists today, versus trying to implement the tools designed in prior ecosystems or prior technology stacks.
In reality, older business intelligence platforms are expensive for mid-market companies. Especially in media and events where the data is complex and lives in multiple layers of platforms. Large organizations can afford to invest in business intelligence solutions, whereas smaller companies cannot. However, that has changed in recent years, in part due to SaaS companies and the availability of products in the market.
The decision of which tools to use is where we see many companies go wrong. They are more likely to purchase or hire the well-known name used by enterprises without understanding the implications and the level of support required to implement those tools. As a result, many companies get stuck and end up with failed implementations of powerful tools that are simply not the right fit.
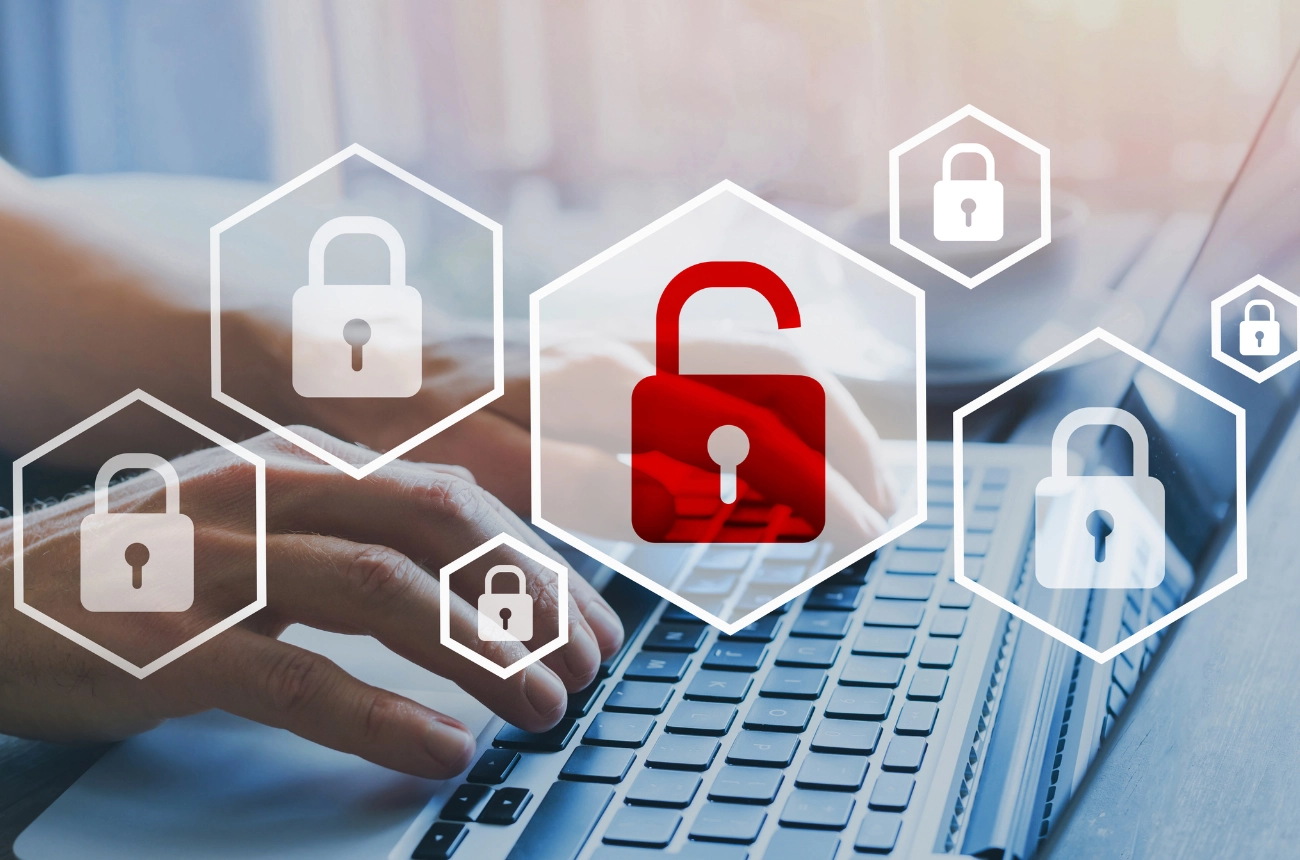
Operationalizing data in your organization
One of the biggest pillars of successful data monetization is democratizing data. It has to be operationalized and part of the daily flow of work.
One of the assumptions when InsightOut, and now Insightify, was developed is users are not data scientists, not technically trained, and not experts in determining the right key performance indicators (KPIs), or what to do with the data presented to them. Users want the same user-friendly experience in their business tools as they have in their personal tools.
InsightOut was built to simplify the presentation of data and make it less like a dashboard and more like an application. We wanted to strike a balance between flexibility and simplicity. The platform needed to give end users the data they need, when needed, and in a format, they can easily understand and use.
Many analytics solutions have failed because they simply provide a few charts and graphs without solving the automation problem or giving users the necessary answers.
When we built Insightify, we focused on a handful of visualizations business users and executives commonly use: , , and intelligence. These visualizations are highly customizable, allowing users to slice, dice, and display the data in a way that automates data preparation and report generation while providing all the necessary data points.
The level of adoption of InsightOut has been extremely high within the companies that have implemented it, and it has become one of their most popular tools for day-to-day use. The need for exporting data to Excel has significantly decreased compared to other tools that fall short of meeting customer needs.
The future of data science in business
Over many discussions with clients, Chad has discovered that the role of data science will change. The change is in part due to advances within AI, or the capabilities to interpret data and decipher what's happening within the datasets, which are becoming very powerful. This will inevitably lead to a situation where a business user should be able to log into a system that has pulled in these different datasets automatically, interpreted the data, and is giving you the outliers or the trends or the specific data points you should be paying attention to today, or for this week, or for this month.
There will always be data scientists at the very edge of the development of analytics where they're looking at coming up with brand new ways of determining what's happening or investigating brand new datasets. A lot of companies who share similar business models should and will have the predictive models automated to a certain degree ten years down the road. In the past, if a middle-market company looking to become an enterprise-level company, they would go out and hire engineers to stitch together a bunch of data. Chad doesn’t believe that will happen going forward. He feels the applications will become exceedingly powerful to help enable business users as they intend to make use of this data.
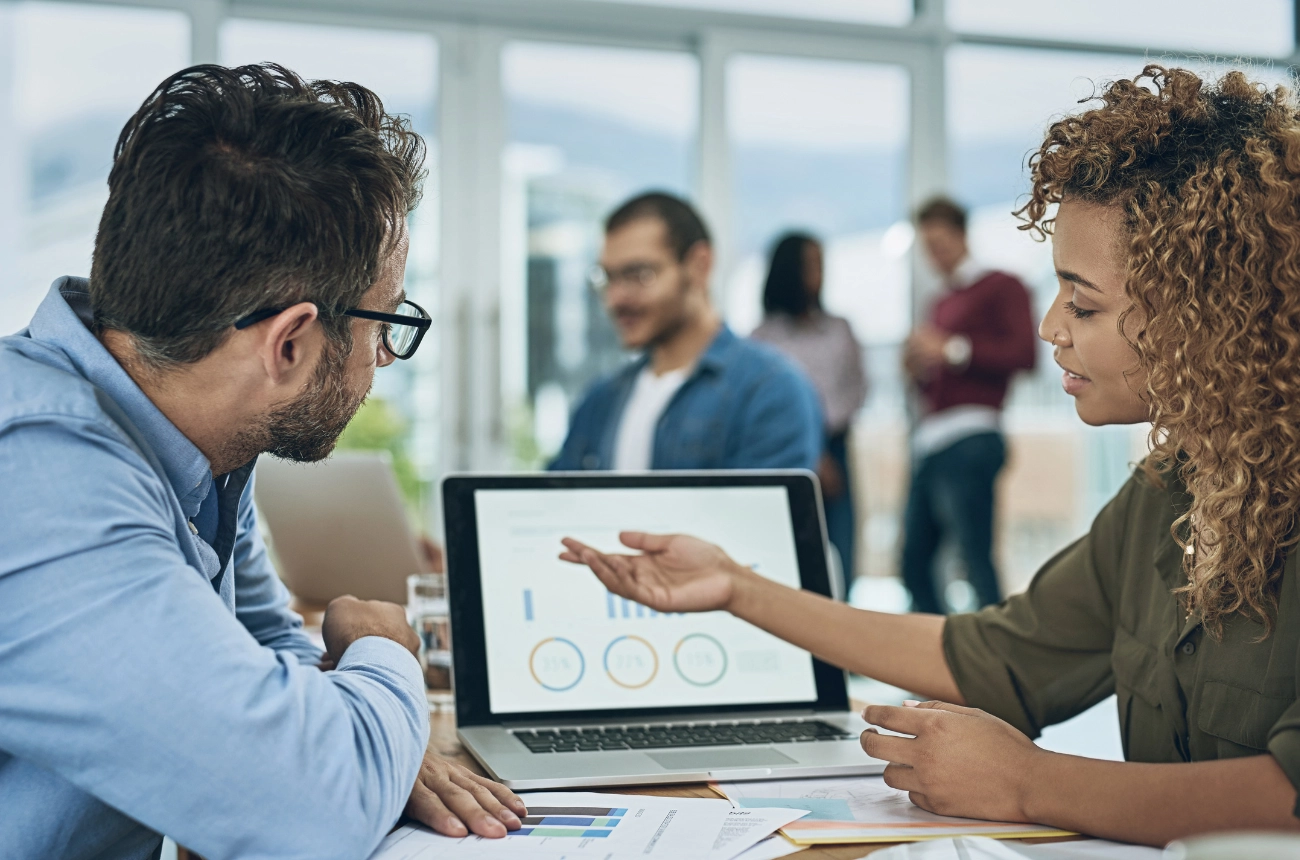
Becoming a data-driven organization
A shared problem across all businesses doesn't matter what type, is that becoming data-driven is a critical imperative if you're going to succeed and thrive in the future. However, it requires financial capital, time commitment, leadership commitment, persistence, and in most cases, significant culture and organizational change. Many times businesses are unable (or unwilling) to justify this level of investment. One of the areas H2K Labs does is help customers define success, identify the business value, and ensure it's measured across the journey. According to Chad, businesses need to start small to become a data-driven organization.
The problem with these types of transformations will only happen and often only start from the executive level. The CFO, CEO, and that level of the executives within the business have to lead change, and they are typically the ones who push transformation, although they are also the ones who might not know or may know the least in terms of technically what's available and what's possible for them to do within their business as it relates to data.
The view is I want to become data-driven. I wanna aggregate this data, or I want to get to some sort of single source of truth. The reality is there are thousand different systems, the team is telling them the data is a mess and not clean. So the perception becomes this is going to be a giant lift, which has to be a giant lift, but it really doesn't. If you approach the goal with that mindset, then you're most likely going to fail.
The solution is simple, start small. Identify the key things which need to take place within the business on a monthly, quarterly basis. If you don't have the technology in place, you're probably doing that manually today. If you're doing that manually, there's a clear ROI to automate that. If you're not automating that, you will be behind very quickly.
You start very small, and you automate what you can within the existing reports. Even within those, you must have a certain eye toward what's worth automating. Then you've alleviated work from your team so they can see the value. You have a working solution that's answering some of the questions you are otherwise getting from manual exercises, and you start to generate some buy-in.
Once you've accomplished the first goal, the team can see a little bit more about what's possible, and they will start asking other questions as to what could come next. And if you take those iterative steps, one-month, two-month types of projects that should get a result at the end of those and you deliver those, then you build that internal support, you clear the ROI threshold, and you can continue to build on each win.